Note
Go to the end to download the full example code
Examine and manipulate stimulus power¶
This shows how to make stimuli that play at different SNRs and db SPL.
import numpy as np
import matplotlib.pyplot as plt
from expyfun.stimuli import window_edges, read_wav, rms
from expyfun import fetch_data_file
print(__doc__)
Load data¶
Get 2 seconds of data
data_orig, fs = read_wav(fetch_data_file('audio/dream.wav'))
stop = int(round(fs * 2))
data_orig = window_edges(data_orig[0, :stop], fs)
t = np.arange(data_orig.size) / float(fs)
# look at the waveform
fig, ax = plt.subplots()
ax.plot(t, data_orig)
ax.set(xlabel='Time (sec)', ylabel='Amplitude', title='Original',
xlim=t[[0, -1]])
fig.tight_layout()
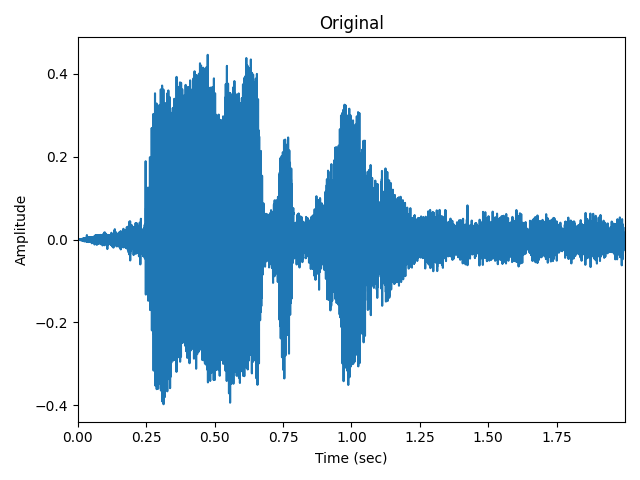
2023-07-25 19:08:46,777 - INFO - Read WAV file with 1 channel and 414951 samples (format int16)
Normalize it¶
expyfun.ExperimentController
by default has stim_rms=0.01
. This
means that audio samples normalized to an RMS (root-mean-square) value of
0.01 will play out at whatever stim_db
value you supply (during class
initialization) when the experiment is deployed on properly calibrated
hardware, typically in an experimental booth. So let’s normalize our clip:
0.08416927712570585
0.009999999999999998
One important thing to note about this stimulus is that its long-term RMS (over the entire 2 seconds) is now 0.01. There will be quiet parts where the RMS is effectively lower (close to zero) and louder parts where it’s bigger.
Add some noise¶
Now let’s add some masker noise, say 6 dB down (6 dB target-to-masker ratio; TMR) from that of the target.
Note
White noise is used here just as an example. If you want continuous
white background noise in your experiment, consider using
ec.start_noise()
and/or
ec.set_noise_db()
which will automatically keep background noise continuously playing
during your experiment.
# Good idea to use a seed for reproducibility!
ratio_dB = -6. # dB
rng = np.random.RandomState(0)
masker = rng.randn(len(target))
masker /= rms(masker) # now has unit RMS
masker *= 0.01 # now has RMS=0.01, same as target
ratio_amplitude = 10 ** (ratio_dB / 20.) # conversion from dB to amplitude
masker *= ratio_amplitude
Looking at the overlaid traces, you can see that the resulting SNR varies as a function of time.
colors = ['#4477AA', '#EE7733']
fig, ax = plt.subplots()
ax.plot(t, target, label='target', alpha=0.5, color=colors[0], lw=0.5)
ax.plot(t, masker, label='masker', alpha=0.5, color=colors[1], lw=0.5)
ax.axhline(0.01, label='target RMS', color=colors[0], lw=1)
ax.axhline(0.01 * ratio_amplitude, label='masker RMS', color=colors[1], lw=1)
ax.set(xlabel='Time (sec)', ylabel='Amplitude', title='Calibrated',
xlim=t[[0, -1]])
ax.legend()
fig.tight_layout()
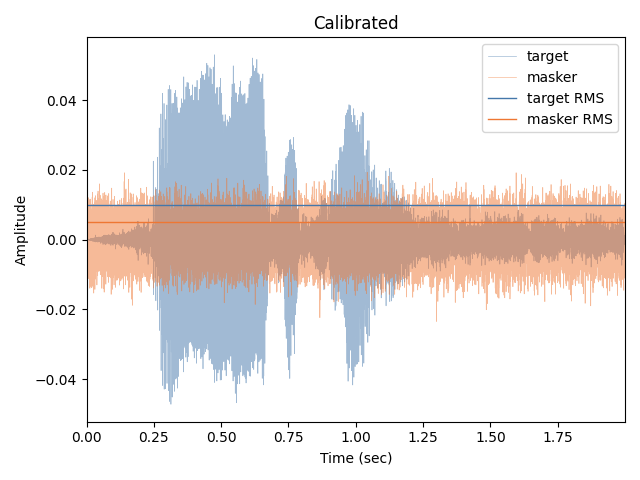
Examine spectra¶
We can also look at the spectra of these stimuli to get a sense of how the SNR varies as a function of frequency.
from scipy.fft import rfft, rfftfreq # noqa
f = rfftfreq(len(target), 1. / fs)
T = np.abs(rfft(target)) / np.sqrt(len(target)) # normalize the FFT properly
M = np.abs(rfft(masker)) / np.sqrt(len(target))
fig, ax = plt.subplots()
ax.plot(f, T, label='target', alpha=0.5, color=colors[0], lw=0.5)
ax.plot(f, M, label='masker', alpha=0.5, color=colors[1], lw=0.5)
T_rms = rms(T)
M_rms = rms(M)
print('Parseval\'s theorem: target RMS still %s' % (T_rms,))
print('dB TMR is still %s' % (20 * np.log10(T_rms / M_rms),))
ax.axhline(T_rms, label='target RMS', color=colors[0], lw=1)
ax.axhline(M_rms, label='masker RMS', color=colors[1], lw=1)
ax.set(xlabel='Freq (Hz)', ylabel='Amplitude', title='Spectrum',
xlim=f[[0, -1]])
ax.legend()
fig.tight_layout()
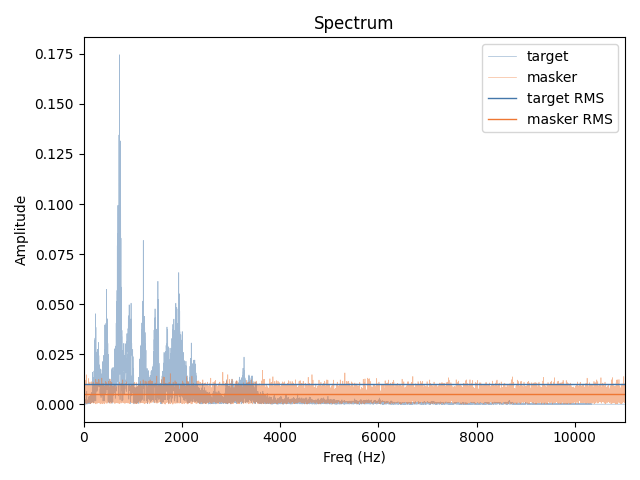
Parseval's theorem: target RMS still 0.009999773251761586
dB TMR is still 5.99988977063175
Total running time of the script: ( 0 minutes 0.745 seconds)